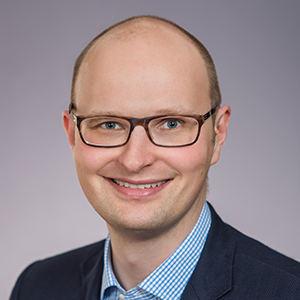
19 Dec Interview with Sebastian Kronmueller of Siemens Healthineers
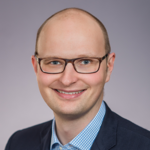
Sebastian Kronmueller incubates startup businesses within Healthineers with a focus on novel molecular applications. His current incubation businesses include the Siemens Healthcare Laboratory in Berkeley, California and NEO New Oncology in Cologne, Germany. Previously he led the execution of strategic initiatives as Head of Special Projects for Siemens Healthineers, playing an instrumental role in shaping the strategy of Siemens Healthineers in preparation for the IPO planned in 2018. Before this role he led a project management team for companion diagnostics and software development and was responsible for the carve-out and divestment of the Healthineers Microbiology business. Read his full bio.
Interview with Sebastian Kronmueller of Siemens Healthineers
Q: Artificial intelligence (AI) techniques have sent vast waves across healthcare, even fueling an active discussion of whether AI doctors will eventually replace human physicians in the future. Do you believe that human physicians will be replaced by machines in the foreseeable future? What are your thoughts?
A: Human physicians will certainly not be replaced by machine learning or AI technologies. There is a lot of hype around the topic of applied machine learning technologies, but the reality of what the models can do is quite different from the popular imagination. AI tools are mainly great for automation of repetitive tasks, pattern recognition – especially in images, and targeted information retrieval from large multidimensional databases. So, they can support physicians for a particular diagnosis (as e.g. demonstrated for diabetic retinopathy) and help them automate parts of their daily live, maybe even become more efficient with the growing bureaucratic burden posed by EMR systems, but they will not replace them or other qualified healthcare personnel in the foreseeable future. Navigating the subtleties of disease and treatment will stay a human domain, but hopefully AI can support to make the lives of both the physician and the patient easier and opens up the possibility of spending more time on core tasks like the important patient-healer relationship.
Q: Can you provide some use cases that have already successfully demonstrate the value of AI/Machine Learning in healthcare?
A: I personally see the greatest value in use cases where we achieve either direct operational improvements or leverage AI to help diagnose disease better. A good operational use case is the use of AI in Siemens Healthineers new Atellica solution to automatically identify the type of sample tubes that have been loaded into the system. It takes a photo of the various sample tubes, uses a pre-trained AI system for identification of their dimensions and automatically configures the system to process them optimally. Great operational application.
Identifying diabetic retinopathy via a pre-trained machine vision system is a good example for a diagnostic application. Another impressive diagnostic application is to use ML to understand changes in the immune system due to cancer. At Siemens Healthineers we also have a variety of strong diagnostic applications, for example automated segmentation and quantification of medical imaging information and the use of machine learning for multi-marker based in-vitro diagnostics. And we currently are working on providing a “digital twin” to physicians which integrates all relevant patient data to aid in diagnosis and treatment. I will talk about this at the conference.
Q: What areas in healthcare will benefit the most from AI/Machine Learning applications and when will that be?
A: My hope is that AI will make physicians lives much easier in the near term. Prepopulating forms in EMR systems, helping with specific diagnoses and reducing the number of individual tasks needed to move a patient through clinical pathways will be great improvements. We will see these tools moving into clinical practice gradually in the next years. The other area in which already sees tremendous benefits is research. AI/ML systems can help with the complex task of patient stratification, identification of novel therapeutics and biomarkers and in general support our understanding of human health. The introduction of AI here is also a gradual process over the next years, not something that will happen overnight.
Q: What are some of the challenges to realize AI/Machine learning in healthcare?
A: In my opinion, all really successful applications of AI/ML are based on real, functional mechanisms that are easier to grasp via the technology. An example from Healthineers is the virtual heart as part of the digital twin. It models the electrical pulses when the heart is beating – a real world, functional mechanism – and helps to understand irregularities. To successfully apply AI we need to improve our understanding of these functional mechanisms, and the way to do this properly is traditional scientific research. So one challenge to realize AI/ML in healthcare is finding the right research results where AI/ML can have a positive impact.
Another challenge is the fact that Healthcare systems already use many, many legacy IT systems which we are already struggling to manage and maintain. In a sense, AI/ML can be seen as an IT technology like relational databases – it enables new applications, but requires strong systems around it to be managed properly. So to make AI work, we have to find a way to upgrade these existing systems or to build appropriate interfaces. And cybersecurity, already a key topic in Healthcare, will become even more important to protect the vast amount of data we’ll be capturing to make AI effective.
Finally, I don’t think the current regulatory and reimbursement mechanisms are adequate to capture many of the most recent advancements. For example, having a computer vision enabled diagnosis is not an IVD and doesn’t have a reimbursement code, but de-facto can be as accurate and clinically relevant as a biomarker driven blood test. We need to make sure we differentiate clearly here between value-added applications and noise, but for the former we need to have clear processes in place how to achieve regulatory approval and adequate reimbursement from CMS.
Q: How close are we with successfully using AI for the purpose of mining big data?
A: I think that is another one of the misunderstandings about AI and “big data”. It is extremely hard to mine big data successfully and to distinguish between signal and noise. If a data set is big enough it is always possible to find many, many correlations which seem meaningful – but they are just noise, the functional mechanism I’ve mentioned before is missing and they cannot be meaningfully generalized. I don’t think AI/ML will change that. When it comes to understanding big data I believe in the strength of classical scientific methods to build understanding and enable interpretation.
Once this is available, e.g. by understanding inflammatory reactions in organs due to pathogens, it is possible to use AI/ML to screen large amounts of data, in this case images, to identify typical patterns. But the understanding comes first. At Healthineers we have gigantic data lakes – over 300 million curated clinical datasets – but we follow the logic of building understanding first, then applying AI to apply the insights to data and then moving towards clinical applications.
Q: What is your outlook or vision for use of AI/Machine Learning in healthcare?
A: I think it will help physicians, healthcare professionals and researchers to perform their job more efficiently, will help make technology supportive instead of distracting and improve diagnostic accuracy and treatment across the board. But it will be based on real insights which we’ve gained by traditional science, and the change will be gradual via many small improvements over time, not sudden and holistic. I think of it more as a technology like relational databases – huge impact for every single human being on the planet, but it happened over time and in parallel to many other technological advances like the internet. The same will be true for AI.
Q: Is there anything you would like to share with the PMWC audience?
A: For me PMWC is always a great opportunity to learn from many brilliant people. So I’m looking forward to many good discussions and presentations! If you see me at the conference and would like to talk, please don’t hesitate to approach me.