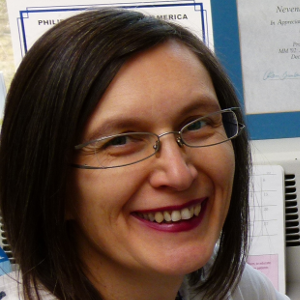
14 Dec Interview with Nevenka Dimitrova of Phillips Healthcare
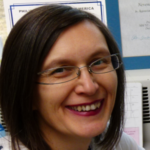
Nevenka Dimitrova is the Chief Technology Officer at Philips Oncology Informatics. With her technology passion and intrapreneurial mind, she is known for contributions in genomics signal processing, AI, and healthcare informatics. Nevenka is committed to bring genomics into the world of practicing clinicians to add precision and clarity – through a new array of cloud and clinical informatics technologies, continuous learning systems and automated reasoning. Her bibliography contains over 140 technical and scientific articles and has over 60 issued patents, and has been given the Gilles Holst award – the highest science and technology peer recognition prize at Philips. Read her full bio.
Interview with Nevenka Dimitrova of Phillips Healthcare
Q: Artificial intelligence (AI) techniques have sent vast waves across healthcare, even fueling an active discussion of whether AI doctors will eventually replace human physicians in the future. Do you believe that human physicians will be replaced by machines in the foreseeable future?
A: No, I believe that human expertise and clinical judgement are essential for foreseeable future and not replaceable by AI. Medicine is about helping people through insight and based on ethical principles, which are high bars for machines at the moment. AI is a technology and should be in service of clinical experts, and when we talk about ethics this is an orthogonal dimension that goes beyond technology having legal and societal implications. Certain aspects of the work will be replaced by machines, where tasks can be more automated – and this is exactly what we are doing in Radiology Informatics with our Illumeo product.
Q: What are your thoughts?
A: In my mind the main question is how to use AI to amplify the clinical expertise and experience of humans. Machines and humans can co-exist where AI can assemble and track all of the necessary information– however, the power of insight and exercising judgement is still within the human experts. Autonomous learning and connecting the symbolic side of AI with machine learning will take some more time.
AI needs to get more advanced to recognize ways to improve performance and to focus on adaptivity, and overcome current obvious drawbacks like brittleness and inability to deal with missing information or new scenarios. Many of the current algorithms are not very adaptive, these are algorithms that are only fine tuned to perform classification tasks, and they don’t provide the necessary explanations how the classification is performed. And this is not intelligence, it’s a simple pattern recognition – this is what I call the first wave
While our enthusiasm for true AI is pardonable, I anticipate that the next wave in AI should tailor medical domain specific unsupervised learning methods – in a helpful and unobrustive manner, how to compare and find similar patients, how to spot real unusual patients in addition to supervised machine learning.
Connecting the dots in a continuously learning system has been the dream of AI for decades. What has been lacking is the discipline of creating self-learning frameworks – that would be the third wave.
Q: Can you provide some use cases that have already successfully demonstrate the value of AI/Machine Learning in healthcare?
A: Diagnostic applications that include pathology and radiology imaging have already relied on machine learning for quite some time. In radiology, Philips has been active in radiomics since the 90’s in what was back then termed CADx – computer aided diagnosis. We introduced Illumeo, software that uses adaptive intelligence in Radiology, tailored workflow experience backed up by an intelligent engine that reads and analyzes image tags, and an image recognition system; allowing for robust hanging protocols that radiologists can rely on. Recently we introduced a platform capable of hosting a wide array of AI algorithms that enable genomics and NLP analysis for clinical and research purposes. We published an algorithm for information flow and network analysis that uncovered important genes responsible for platinum resistance in ovarian cancer. Our OncoSignal analysis assessing tumor-driving signaling pathway activity relies on advanced Bayesian technology. We continue to innovate on various tumor response assessment algorithms in radiomics. In computational pathology, we have introduced an algorithm called TissueMark that uses deep learning for region of interest detection and cellular profile estimation in digital whole slide images.
As part of our IntelliSpace Precision Medicine platform, we introduced an application for finding similar patients – if the most relevant similar cases are brought up to the doctor, it is quite straightforward for them to learn from the past experiences of similar patients.
Q: What areas in healthcare will benefit the most from AI/Machine Learning applications and when will that be?
A: The first instance I see in driving Precision Medicine anyway, however, we need to have enough validation studies to convince both the clinicians and the payers. Oncology is poised to benefit from AI / machine learning now – even with the supervised algorithms for predicting outcomes based on response to pharmaceutical agents or combination treatments with radiation treatments. Expanding this to pediatrics, rare diseases would be next. In acute care predicting sepsis and onset of a hospital acquired infection would be next. In all of these domains AI is helping to improve efficiency and outcomes.
Q: What are some of the challenges to realize AI/Machine learning in healthcare?
A: AI functions quite well in the supervised learning mode, when there is enough clean and correctly labeled data available. Accessibility to large high quality trusted data sets with all associated diagnostic information, prescribed vs. received treatments and outcomes in addition to lifestyle data is paramount to having a good learning environment for a certain hypothesis. To collect such information with the proper data access frameworks, while preserving all the privacy and security of the information is a formidable challenge.
Q: What are the products and/or services Philips offers/develops in the AI/Machine Learning sector? What makes Philips unique?
A: We have a Precision Medicine platform that offers a comprehensive set of workflows for creating a complete patient picture as well as providing clinical decision support along the diagnosis, treatment and monitoring phase of the patient journey. The platform serves to capture clinical evidence and enable knowledge management along with judicious combination of predictive analytics tools. The therapeutic strategy is created by clinical experts with our tools for automating the authoring, and delivery of clinical decision support with the curated clinical pathways. We enable asynchronous data gathering and asynchronous multidisciplinary approach to match against the pathway decision trees. A patient with non-small lung cancer may be treated differently depending on whether there are targetable genomic mutations present and whether the patient has had prior immunotherapy, but also based on clinical factors such as performance status, comorbidities, and patient preferences alongside molecular features of the tumor and immune-system markers. The eligibility for immunotherapy and the specific type of immunotherapy is not established by an algorithm; it is established by clinicians who have reviewed the published clinical evidence (including the clinical trials and the drug labeling, toxicity and side effect profiles), discussed and debated before establishing the clinical pathway. In certain circumstances, clinical trial matching becomes the prefered option – based on complex information form the trial description and from the patient record.
What makes us unique is our long tradition in diagnostics, signal processing and AI, and the wealth of knowledge on how we approach technology integration. Furthermore, we recognize that cancer care is decentralized, being delivered through a combination of larger academic centers and cancer networks. We have partnered with the key opinion leaders such as Dana-Farber Cancer Institute, Boston Children’s Hospital, and Leiden University Medical Center – as all stakeholders need to trust that they can get the same high quality care from any provider and that the information relies on evidence-based standards. For a complex area such as oncology, we created a platform to cohesively integrate human expertise and knowledge derived from long-standing research and a process for creating a consensus. This is preferred and orthogonal to a straightforward AI approach that can rely only on predictions and probabilities. AI algorithms can give probabilities while human experts need decisions to be supported with published clinical evidence which is captured over many discussions for weighing in the pros and cons.
Q: What are the short-term challenges that Philips and its peers are facing?
A: Despite all the interoperability standards it is quite hard to enforce a standardized way of capturing data and leveraging the past experiences of the patients for improving outcomes of the new patients. Well established ethical frameworks around leveraging past patient experiences and data access are also lacking despite many efforts.
Q: What is your role at Philips and what excites you about your work?
A: As a CTO for Oncology Informatics and Genomics I have a unique role to bring most of the AI technologies within the hands of practicing clinicians. I have spent most of my professional life in AI, and only now I feel that from the leaves we can start to see the forest. I believe in creating an environment where physicians can trust the data and AI methods, as well as collaborate with each other. We have now the platform to include the most advanced algorithms for similar patients, for radiomics, pathomics and genomics. I am excited about the fusion of these ‘omics with AI to elucidate information from the organ level all the way to single cell genomics – which I anticipate will change the way we look at cancer in the next few years.
Q: Is there anything else you would like to share with the PMWC audience?
A: Achieving the AI in medicine aims: getting better outcomes, enhancing the patient and healthcare provider experience and decreasing cost – is a very complex task. AI needs to help in the true digitization – to augment humans rather than replacing humans. Adaptive aspect of AI is a key point so that technology can be like a personal assistant, AI needs to be natively integrated in the workflows of the doctors, embedded to deliver help at the point of care. Most essential is to truly leverage the medical history, comorbidities, response to past treatments for an individual patient in the context of the insights from similar patients on a global scale.