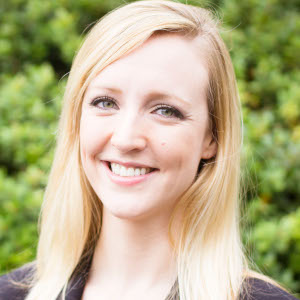
13 Sep Q&A with Shannon Muir, Science Officer, Governor’s Office of Planning and Research
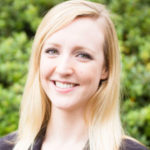
Dr. Muir co-leads the California Initiative to Advance Precision Medicine. In this role, she works with the Precision Medicine Advisory Council and the Governor’s Office in the selection and management of grants for precision medicine demonstration projects. Her current portfolio consists of projects that aim to address health disparities in cancer prevention and treatment. Dr. Muir also manages strategic relationships with State entities and other stakeholders including community groups, academics, and private sector partners. Read her full bio.
Interview with Shannon Muir, Governor’s Office of Planning and Research
Q: What are the overarching goals of the California Initiative to Advance Precision Medicine (CIAPM) and can you give us an idea of the types of demonstration projects you have undertaken?
A: The goal of the Initiative is to maximize the use of the California’s enormous resources in healthcare delivery, scientific and medical research, and data and technology development to improve human health through precision medicine. The Initiative seeks to fund research that has a high potential for tangible benefits to patients in two to five years, so the projects have been very translational. The Initiative also seeks to address health disparities; for example, this year we funded three teams that are all looking at cancer disparities in the Latino and Latina population compared with the rest of the state.
Q: One of the projects used AI to speed the time to diagnosis for brain emergencies. Can you describe this project, why it’s important and how its success has led to a “first” in the neurologic imaging area?
A: The Artificial Intelligence for Imaging of Brain Emergencies project was led by Pratik Mukherjee, Esther Yuh, and their team at UCSF and UC Berkeley, and encapsulates many of the goals and values of the Initiative. The team was eager to improve the time to diagnosis for neurologic emergencies including hemorrhagic stroke, aneurysmal subarachnoid hemorrhage, and traumatic brain injury, the last of which disproportionately effects underserved communities. In these cases, time to diagnosis can mean the difference between life and death. The current standard of care in a suspected case of a neurologic emergency is to perform a head CT scan which must then read by a radiologist. Dr. Mukherjee’s team trained an AI system to diagnose brain hemorrhages with the same rate of accuracy as board-certified radiologists, but much more quickly. The AI system can also measure the extent of bleeding. These quantitative “imaging biomarkers”, if adopted by the FDA, would be the first objectively measured indicator of neurologic emergencies. The system Dr. Mukherjee’s team developed also eclipses current practice because it can make clinically important distinctions between different types of hemorrhage and accurately display the locations of each type in different colors, as opposed to assigning scans as either normal or abnormal. This detailed information is valuable not only to radiologists but also to Emergency Department physicians, stroke neurologists, neurosurgeons as well as to patients and their families.
Q: What happens to the data that is generated from the projects that the California CIAPM undertakes, how will it be used, and how are the issues of data privacy and interoperability being handled?
A: We encourage our teams to upload their data to public repositories so that their utility can continue beyond the scope of the CIAPM-funded project. One successful example is the California Kids Cancer Comparison (CKCC) by David Haussler at UC Santa Cruz. His team uploaded all of their data to a publically available pediatric tumor compendium as part of the Treehouse Childhood Cancer Initiative, which is housed in the UCSC Genomics Institute. Likewise, the brain emergencies team from the previous question uploaded their data for Federal Interagency Traumatic Brain Injury Research (FITBIR). However, almost every team identified data acquisition and sharing among the main barriers they faced over the course of their project. For instance, one of our teams was originally unable to work with a private research facility after that facility transitioned away from allowing authorized users to download data to their own servers toward a model which required computation to be done within facility’s own hosted platform. The price of the new data sharing model would have made acquiring the data prohibitive, except for the fact that the team was able to negotiate by framing their work as a collaborative pilot project.
Several teams commented that the development of standardized guidelines and regulatory requirements for data use would stimulate increased data sharing and make the data more accessible to researchers by reducing the resources spent on acquisition. As researchers experience the growing pains of dealing with interoperability and sharing, it would be highly advantageous if they were able to capture and publish lessons learned that are specific to data sharing.