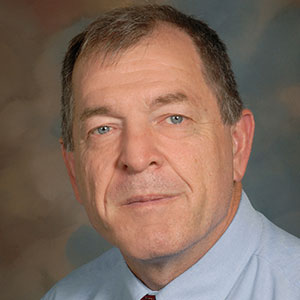
06 Sep An interview with Stanley Huff, Chief Medical Informatics Officer, Intermountain Healthcare
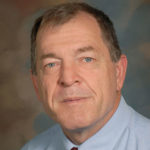
Dr. Huff is the Chief Medical Informatics Officer at Intermountain Healthcare.
He has been working in the area of medical vocabularies and medical database architecture for the past 25 years. Dr. Huff currently is a fellow of the American College of Medical Informatics, a co-chair of the LOINC Committee, a co-chair of the Health Level 7 (HL7) Clinical Information Modeling Initiative (CIMI), the chair of the Healthcare Services Platform Consortium (HSPC), and a past chair of HL7. Read his full bio.
Interview with Stanley Huff, Intermountain Healthcare
Q: Genomics, Digital Health, Big Data, and Artificial Intelligence are some of the newest technologies/fields that are reshaping medicine and healthcare. • How will these technologies impact healthcare and in particular individualized medicine? • What is it that we can realistically expect from these technologies to improve healthcare and in what timeframe? • What is still required to take advantage of the full potential of these technologies?
A: What we can expect from the new AI technologies is new knowledge, to discover things that we do not yet know. This will be additional knowledge that helps us understand how to treat patients, and help them get better and improve the quality of healthcare that we provide to them. We are having an impact today, but we will see an increasing impact as people implement new knowledge discovery tools. We are not waiting for a day when we can say, well, we’re there, we now have all the knowledge that we need. We have got to do everything we can with the knowledge that we have now.. I believe it is a journey. For example, we might learn about a new association between a person’s genetic makeup and the way they metabolize a specific drug. This will allow us to use that newly attained knowledge to not prescribe this drug if the patient would not be able to effectively utilize it. Therefore, this week we will know about one drug and in a year from now we will know more about 20 additional drugs and ten years from now we will know more about 1,000 drugs. Hence, over time the knowledge increases – it is basically a limitless number of things that we still need to learn and that will help us take better care of patients.
In terms of expectations we have for these technologies:
1. Acceleration of knowledge extraction: The algorithms are fairly tolerant when it comes to missing data or inaccurate data and they can overcome the problem of dirty data. This is achieved by having data from a large number of people that we can analyze. This allows us to overcome the errors that impact small data sets as to compared to when we can look at really large numbers of people. At the same time, we want to do everything we can to make sure the data is as clean as possible to begin with.
2. Access to the raw/clinical data: There are lots of kinds of data that we access to, but there are lots of important data that is either never entered into the clinical record, or it is hidden in narrative content. Lots of things are not noted in clinical systems that would be valuable to know if we wanted to answer certain questions. We need higher consistency in data representation and data collection which overall would lead to faster learning. I would expect new technologies to address this component more efficiently.
Q: Data science is a huge driver of healthcare and several of the sessions of the AI track, the track you are chairing, touch upon the importance of data science. 1. Where do you see the biggest impact of data science in healthcare? 2. How will it help with cost reduction? 3. How will it contribute to a value-based care system?
A: The foundation for good medicine is the ability to create new knowledge that is based on reliable, consistent, and computable data – to me this is what data science is all about. It is about the semantics (meaning) of the data. If you consider all of the aspects of data science
• It is not only about the analytics, but it is also about data governance.
• It is about who can access and use the data.
• It is about how the data is represented.
Those elements are essential, in order to be able to apply artificial intelligence and deep learning algorithms to learn and advance what we are doing.
In terms of cost reduction: Newly acquired knowledge can improve the quality of care that an organization is providing. For instance, if we are looking at the genetics of a particular tumor the data may allow us to predict whether the tumor is susceptible to certain chemotherapeutic agents that will have a positive impact on the outcome of treatment. If we can use new knowledge to improve outcomes that creates value-based care. Or the decision could be that chemotherapy has no positive impact and therefore one should opt out. In all of these situations, the patients are better off because they do not have to endure the unwanted side effects of the chemotherapy and in addition, the cost of the drug would be eliminated. In a different situation, we might be able to prevent unnecessary actions, such as unnecessary surgery. But of course, the same kind of knowledge can help choose the right drug that actually has an effect in the first place. Basically, patient treatment occurs not based on just the general population statistics, but based on the patient’s individual genetic profile. And this will change over time as we learn more and more, but it is always an improvement and it will always provide better healthcare and it will reduce costs because you are giving people only the things that you know will be effective in this specific patient which means that you are reducing unnecessary cost. Overall the outcome is better care at a fraction of the cost, mostly because treatment is targeted to the specific genetics, age, sex, diagnosis, metabolism and situation of a given patient.
“The foundation for good medicine is the ability to create new knowledge that is based on reliable, consistent, and computable data – to me this is what data science is all about.”
Q: You have been actively involved in helping develop the HL7 FIHR (Fast Healthcare Interoperability Resources) standard for the purpose of exchanging clinical data electronically. • Why were these standards needed – can you provide an example?
A: Yes, there are lots of reasons, not just one reason why FHIR was created. The single biggest motivation was that they wanted to make sharing standard data easier. FHIR was designed specifically to work with the same infrastructure and tools that people are familiar with when developing web applications. FHIR uses RESTful services for accessing data that are used in all of the internet applications that everyone develops. There are a huge set of tools and knowledge people can access for using RESTful services. Therefore, the motivation was to make it easy and simple for people who already know how to develop web applications to share and create interoperability using these RESTful FHIR services.
FHIR is really a definition of application programming interfaces (APIs) for how to exchange data between systems which previously only supported data exchange using older versions of the standards.
I don’t know what the exact metrics would be, but it would be on the order of six months for programmers to understand how the older versions of HL7 standards work and how to set up and configure a system for sharing the data based on say HL7 V2. Using the FHIR APIs, people are now able to do the same work in one to two weeks instead of six months – and this includes setting up the infrastructure for data sharing.
Q: Is FHIR replacing the HL7 standards or is FHIR developed for a different purpose?
A: It is both. FHIR is replacing some of the older standards in certain situations, but FHIR is also being used in a new situations. While HL7 V2.X is almost universally used for moving laboratory data from the testing laboratories into electronic records systems, FHIR is predominantly used to create new interfaces – which is why we at Intermountain Healthcare are doing mostly FHIR for our new development. We have HL7 version 2 interfaces that are doing pretty much everything we need them to do in terms of that kind of simple data transfer. And we will probably just keep using HL7 version 2 in those simple and well-known data transfers.
On the other hand, the HL7 FHIR standards enables the ability, for one health care institution to ask another one in real time for a specific piece of data and receive the specific data in real time. That real time behavior is not well supported by the old versions of HL7 standards. The new standards are now all part of that RESTful architecture which allows me to ask in real time another healthcare system, “what medications were given?” to a particular patient or “what lab data was collected?” for the same patient. Bringing data from multiple systems together in a real time environment via an application such as this one, was not possible in the past. FHIR will predominantly be used in that real time space. So sometimes FHIR will be used where other older HL7 standards were used in the past, but then again, in many situations FHIR is now used in an entirely new way because it creates new capabilities.
Q: Are there some other standards that are needed to make data shareable in support of various applications or is HL7 FHIR already addressing a lot of those demands in the healthcare sector?
A: The best way to explain this is with an analogy. For example, if there was a highway, FHIR would tell you what kinds of vehicles are allowed on the highway and where you can go on the highway, but additional information is needed to explain what is transported in the vehicle. To understand in a computable way what is being carried in the vehicle requires the use of standard terminology. Another analogy is the mail system. If you are going to send a letter, you need to have an address on the letter; you need to have a stamp on the envelope; and you need to have a return address. But then there is another piece of paper sealed inside the envelope that carries the actual information you are trying to communicate the structural part of FHIR is like the envelope and stamp, etc. that allows you to send a letter. But then you need standard terminologies to express the exact meaning of the message, which correspond to the words that you actually put on a piece of paper inside the envelope. And you need the codes to be standardized or the message will be misunderstood. If I send a letter written in English, and the person receiving the letter only understands German, then the receiving party cannot do anything with the content, because they cannot decipher the content. The sender and receiver need to understand a common language or no communication occurs. The exact same concept applies to the sharing of clinical data. The clinical data needs to be in a defined structure but you also need LOINC codes or SNOMED codes to make the exact meaning explicit. You need specific and exlicit standard ways of coding healthcare information so that you can ask very specific questions from the data in the various systems. Only that way will other systems understand what specific question is being asked, and can return the right data or information back to the requester.
Q: The integration of clinical genomics with other clinical and/or patient related data has been notoriously slow over the past years, though we detect a positive trend towards a change. I assume the HL7 FHIR standards will help with accelerating this. • By when can we expect full integration and adoption of genomics data in the clinic? • How can we overcome the challenges of a non-harmonized terminology of medical/phenotype data?
A: Yes, that is a very hard problem, though a solvable problem. This is a space where I do a lot of work. Here are some of the most important things I have to say about this subject:
• Traditionally when people build systems, they are not thinking necessarily as their first priority about the ability to share that information with other systems, especially systems that might be very different from the system they have. Therefore, they create their own local codes that they use to identify data elements, but they are initially just talking to themselves, so the local codes work fine. But as soon as they start trying to talk to another system or put the data into a data warehouse or into a data lake, or they want to share it for patient care or analytics then they really need to use a standard terminology. Talking in an understandable way to an external system is different from using your own internal system. You can do things quickly when you are just talking to yourself. You can just make up your own codes.
• As soon as you want to talk to other people and you want your system to be part of a community which includes sharing information/data and being able to commingle genetic data with other kinds of patient care data, such as laboratory data, history and physical findings a common language is required to facilitate that.
• Unfortunately, we are in a situation where we have gotten into a bad habit of every system that we create has to have their own terminology. For example, when one installs an electronic health records system, a set of starter or standard codes is used. But then people quickly add local variations onto that starter set and begin creating their own local codes. As a result, one does not get the real communication that is required.
We need to agree what standards we are going to use and how exactly we are going to use them. It is not as simple as saying we are going to use LOINC codes or SNOMED. Because even SNOMED allows more than one way to say the same thing within that specific coding system. One can also use different combinations of LOINC codes to describe the exact same thing. We need to put rules and agreements in place amongst those who are sharing data. Which means that when I share someone’s glucose value, we have to agree on exactly which LOINC code we use in that specific situation and which units of measure are going to be used. It should also include agreement about how the number appears with its appropriate number of decimal points for significant digits – all of these sorts of details have to be agreed on. This can be challenging, because the number of things that we have to agree on is huge, but it is finite and doable.
“Not having the same standards in place across systems is a huge barrier to doing analytics efficiently, or to provide patient care, or to share information for use in a learning health system.”
All in all it is a long journey to get to a hundred percent data sharing. There are many folks that are working on various aspects to get this achieved. One of the exciting projects is called LIVID, which stands for LOINC codes for in vitro diagnostic tests. It is a combination of private organizations as well as the FDA – with Michael Waters at the FDA – working on this in concert with a combination of instrument and test kit manufacturers. They are creating complete instrument-based solutions in combination with various test kits that measure a particular analyte (e.g. cortisol). The LOINC codes are linked to the test result which is the output of the instrument/kit. This results in the manufacturer determining what the correct code representation should be for a specific analyte and specimen. This new process changes fundamentally what can happen in terms of standard mappings for laboratory data. It creates the potential for an incredibly more efficient system. It pushes standardization in a new direction: everybody that uses the same test kit will be using the LOINC code defined by the manufacturer of the kit. Eventually, we end up with an entirely uniform representation of the data at the time that it is created. It eliminates a bunch of translations from one coding system to another coding system, or from a local coding system to a standard coding system. Ideally, this approach will be applied to many more areas, not just lab data. Not having the same standards in place across systems is a huge barrier to doing analytics efficiently, or to provide patient care, or to share information for use in a learning health system.
Q: What are you expecting PMWC attendees will walk away with from the PMWC 2020 Silicon Valley conference? • What is the call to action that we as a community should focus on?
A: We need to invest the time to create interoperability so that we can take better care of patients. We need to do something about the 250,000 patients who die every year in the US from preventable medical errors. That should be a real motivation to everyone. So, we need interoperability to be able to share knowledge as executable programs to prevent those deaths and to take better care of patients.
“The call to action is to do something about the 250,000 patients who die every year in the US from preventable medical errors. We need to invest the time and resources to create interoperability so that we can take better care of patients.”